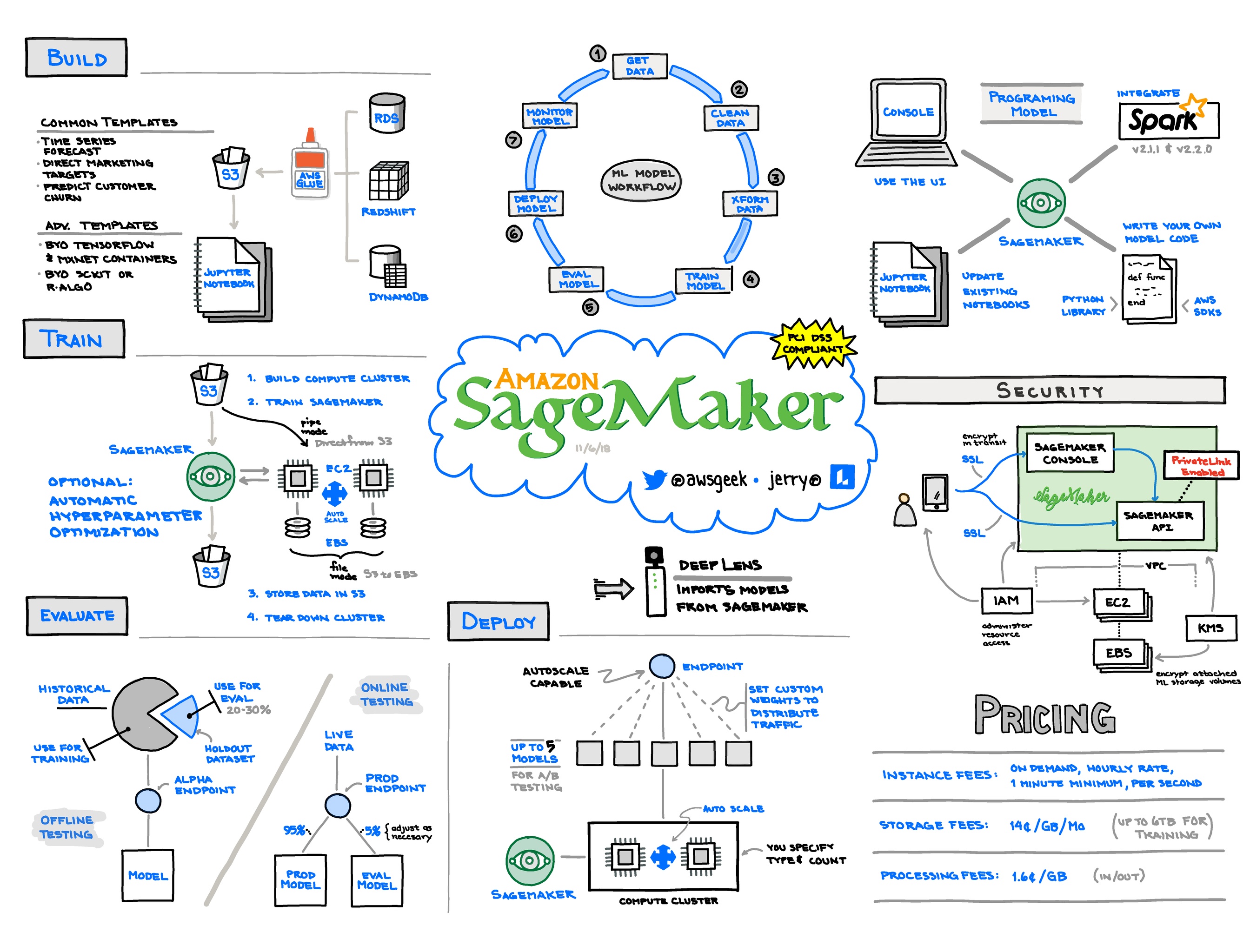
Amazon SageMaker serves as a versatile machine learning platform designed to simplify the process of building, training, and deploying machine learning models at scale. A core component of AWS, SageMaker is designed for professional developers and IT administrators who require a comprehensive machine learning environment that offers robust features and flexibility. This article delves into the technical aspects of SageMaker, exploring its use cases, pricing structure, scalability, availability, and security features. Furthermore, we will evaluate similar services offered by other cloud providers to highlight competitive offerings.
Use Cases
Amazon SageMaker empowers developers with various functionalities suitable for different machine learning tasks. This platform supports the entire machine learning workflow, from data labeling to model deployment in the production environment. SageMaker offers pre-built algorithms that can be used out-of-the-box for tasks such as regression, classification, time-series forecasting, and natural language processing. Developers can also leverage its support for Jupyter Notebooks to prototype and test algorithms using their Python libraries. SageMaker’s real-time endpoints make it seamless to transition from training to deploying machine learning models for real-time inference. These use cases make SageMaker appealing for industries like healthcare, finance, and retail, which require sophisticated data insights.
Pricing
The pricing of Amazon SageMaker is designed to match its broad feature set and scalability. Users are charged based on the components they use, including fees for data storage, compute instances, and data transfer. The cost associated with each machine learning model largely depends on the computational power and time required for training and inference. SageMaker offers a free tier option that includes 250 hours of t2.medium notebook usage per month for the first two months, allowing new users to test its capabilities. Detailed pricing information can be found on the AWS SageMaker pricing page.
Scalability
SageMaker stands out because of its ability to scale, thereby catering to both small datasets and massive data requirements. Its infrastructure can automatically adjust compute capacity to meet the demands of training jobs, which helps in optimizing resource usage and maintaining performance efficiency. SageMaker's integration with Amazon Elastic Container Service (ECS) allows for containerized model deployment, which can be scaled across clusters, meeting the needs of enterprise-level applications.
Availability
Amazon SageMaker is built to deliver high availability, benefiting from the extensive network of AWS data centers globally. It ensures consistent performance and minimizes downtime by employing multiple Availability Zones. This ensures model training and deployment can take place without disruption, providing users with reliable access to their machine learning models.
Security
Security is a top priority for Amazon SageMaker. SageMaker provides comprehensive security features, including network isolation, encryption of data at rest and in transit, and integration with AWS Identity and Access Management (IAM), allowing developers to control access rights effectively. With SageMaker, users can utilize AWS Key Management Service (KMS) for centralized key management, offering an additional layer of security for sensitive data.
Competition
In the landscape of cloud-based machine learning services, several alternatives to Amazon SageMaker are worth considering. Google Cloud offers Google AI Platform, which provides managed services for training and deploying machine learning models. The platform supports TensorFlow, Keras, and many other frameworks, focusing on DevOps integration. Microsoft Azure comes with Azure Machine Learning, a service that helps design and deploy machine learning solutions using a drag-and-drop interface. Azure emphasizes integration with its ecosystem, enhancing the flexibility of model management. Alibaba Cloud's Machine Learning Platform for AI is another competitor, offering solutions catering mainly to the e-commerce and retail sectors. It provides an efficient data processing environment with its machine learning tools and is integrated with Alibaba Cloud's broader suite of services.